With the growing demand for performance in edge computing, the ability to efficiently carry and process large amounts of data at the terminal has become a significant bottleneck for industrial plants and medical applications. With space at a premium, installing high-performance edge servers is the most effective way to reduce operational and maintenance costs by minimizing floor space and simplifying deployment architecture. DFI's ICX610-C621A ATX motherboard with Intel Xeon processors provides reliable performance for AI applications and abundant expansion slots to provide ample bandwidth. Simplifying the deployment of edge computing devices and increasing productivity with the most streamlined architecture.
Industry: Edge Computing / Edge Server
Application: Industrial Automation and Medical Imaging System
Solution: ICX610-C621A ATX Motherboard
Terminologies of Edge Computing
In the industrial and medical sectors, machine vision is the mother of Industrialization 4.0 and an essential part of maintaining efficient productivity. Machine vision is the most common application of artificial intelligence, and the computing devices responsible for machine vision are particularly important in terms of performance. It needs to receive image data from the terminal device without delay, analyze it quickly and accurately and provide feedback, and store the results and record the corresponding images simultaneously.
In the framework of edge computing, this data must be processed and analyzed as close to the terminal as possible, rather than being sent back to the cloud. Software, hardware and data all operate as close to the edge as possible. This not only reduces transmission bandwidth, but also prevents data delays from slowing down productivity and allows for the fastest possible response time to communicate with equipment.
What does computing at the edge involve? Video, sound and sensory data from a variety of devices must be extremely detailed in order to produce accurate results in demanding production lines and medical applications where precision is important. Fine grained content means there is a huge amount of data to handle. In a nutshell, it is difficult to meet end-to-end computing needs with a cloud-based architecture. But deploying a large number of complex computing devices at the end of the line poses a problem in terms of space and maintenance costs. It is only logical that the edge computing server should take on this role, receive data, process it and send it back to where it is closest.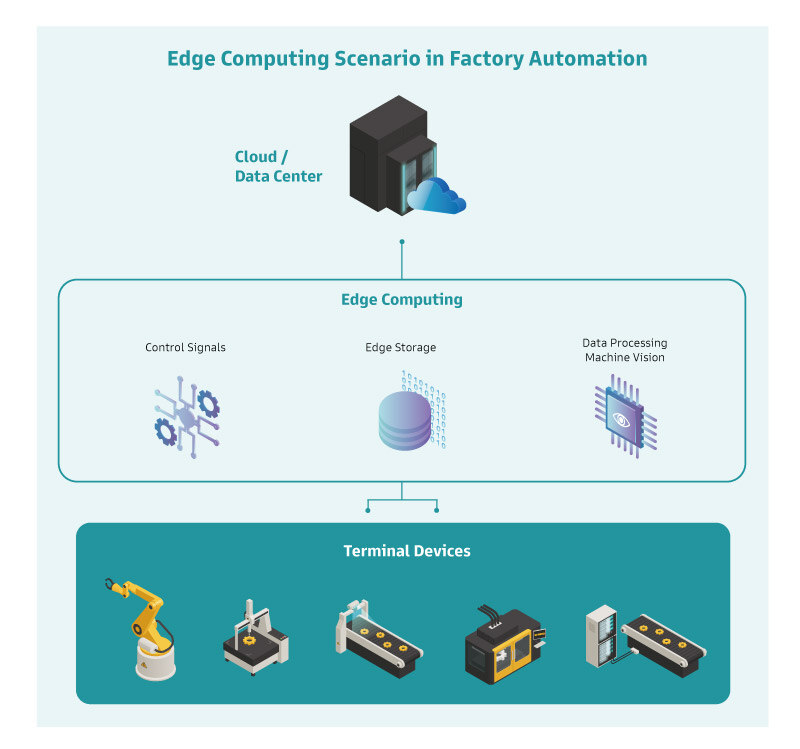
An example architecture for edge computing in factory automation applications. The terminal device communicates with the edge computing server and sends back the data. The server analyzes the data, sends back the corresponding control commands, and stores the data locally and then sends it back to the cloud if necessary. The cloud host should minimize the need for real-time analysis to avoid communication delays.
Critical factors of an edge computing server
An edge computing server needs to have the capabilities of a micro data center and edge cloud, without compromising on processing speed, transfer speed and storage efficiency. The computing focus is on real time and accuracy, and transmission must be low latency. Data access requires sufficient bandwidth and space, which is the specialty of server-class motherboards. DFI's ICX610, for example, combines an Intel Xeon processor to meet the data processing needs of multiple end devices, and an impressive number of internal and external ports to provide ample transmission channels while managing a large number of storage devices, plays an one-stop solution of edge computing. The ICX610 is an Intel Ice Lake platform that supports the third generation Intel® Xeon® processors, which offer outstanding AI computing power and built-in graphics performance. If you were to list three core capabilities, they would be:
- Intel Deep Learning Boost
- Intel AVX-512
- PCIe 4.0 Support
Deep Learning Boost is not new to this generation of Xeon processors, but this technology, based on the AVX512 VNNI script set, has become more powerful as the processors have been updated, resulting in significant performance improvements in both deep learning and visual analytics. In the training phase of AI applications, the performance improvement is as much as 60%. In real-world inference, it is more than 30 times faster than the first generation.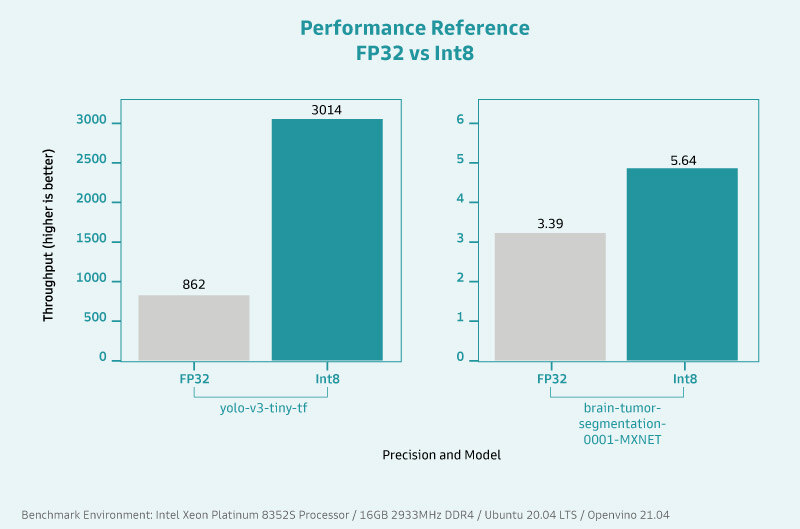
With the optimization of low-precision computing (Int8), the difference between Int8 and FP32 in yolo-v3-tiny-tf is nearly 3.5 times, while brain-tumor-segmentation is 1.6 times.
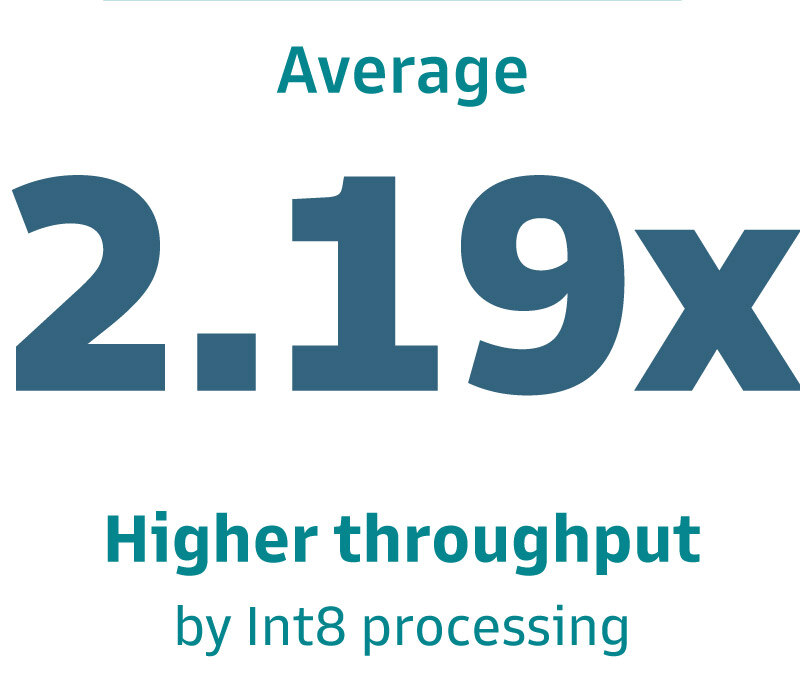
Using more rigorous data for judgement and parsing, VNNI exponentially increases the performance of low-precision computing for AI deep learning and inference. The graph shows the difference in the amount of data processed by Int8 and FP32, with the former being 2.19 times faster than the latter, implying a time speedup of about 45%.
Is that 45% time saving so critical? Imagine that the time taken to identify a product defect on a production line is 25 milliseconds (Note 1), but with nearly half the time saved it will be less than 15 milliseconds. The cumulative effect is that more products can be identified in the same amount of time. And the savings in man-hours and productivity that can be achieved with mass deployment are obvious.
In medical applications, this can also reduce physiological scans or exposure times that can be uncomfortable for the subject.
(Note 1:
The speed of recognition varies according to the complexity of the image and the load on the machine, the values here are purely for comparison purposes.)
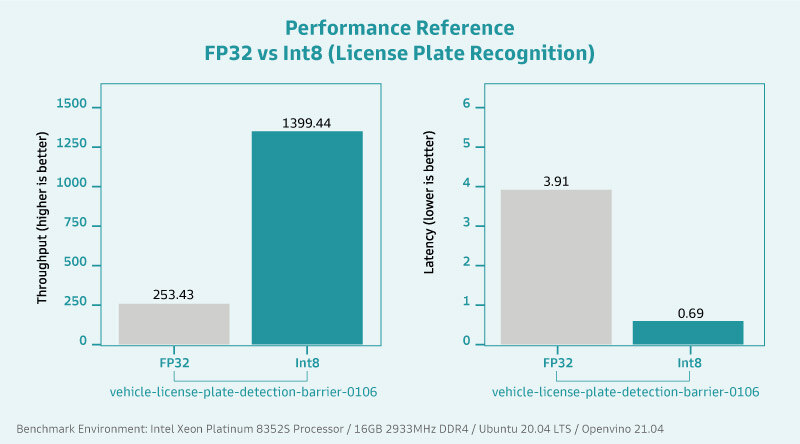
Another scenario fits to edge computing: Smart transportation.
License plate recognition applications in intelligent transportation often require edge servers as a computing node. The faster the recognition speed is, the more immediate the system can respond, and the smoother and easier the control of parking spaces and gates.
Int8 requires less overall storage capacity and less read bandwidth due to its smaller data size, which naturally reduces processing latency and increases throughput. In this application, Int8 has 6 times the throughput of FP32 and nearly one-eighth the latency.
PCIe 4.0 bandwidth leap
PCIe 4.0 provides the maximum bandwidth that a single slot can carry, and when combined with the number of multipliers, the amount of data that can be carried is significant. The ICX610 has three PCIe x16 slots and eight PCIe x8 slots. If you simply count the uncompressed image data that can be processed in one PCIe x16 slot, a single slot can handle two 8K images at the highest sampling frequency (Note 2). This is by far the most stringent standard. And the number of streams that can be handled with compressed, low-flow 4K or Full-HD images is staggering. Combining the above, the ICX610 also adopts an "as many as possible" strategy when it comes to peripheral port placement. There are two 10GbE Ethernet ports alone, and ix SATA and one NVMe slot is packed in while the number of PCIe slots is maximized. The memory utilizes four channels and eight modules to achieve a maximum capacity of 512GB. From data acquisition to computing and storage at the back, all are connected at the highest bandwidth specifications to create a superhighway of edge computing.
ICX610-C621A Spec
- 3rd Gen Intel® Xeon® Scalable Processor Family
- 8 ECC-RDIMM up to 512GB
- 2 x 10GbE
- IPMI OOB Remote Management
- Single Display: VGA resolution up to 1920x1200 @ 60Hz
- Multiple Expansion: 3 PCIe x16, 2 PCIe x8, 1 x M.2 M key
- Rich I/O: 2 Intel GbE, 1 Dedicated IPMI, 2 COM, 5 USB 3.1 Gen1, 5 USB 2.0
- 15-Year CPU Life Cycle Support Until Q2' 36 (Based on Intel IOTG Roadmap)
(Note 2:
7680x4320 resolution, 60 frames per second, 16bit color depth, 4:4:4 color sampling.)
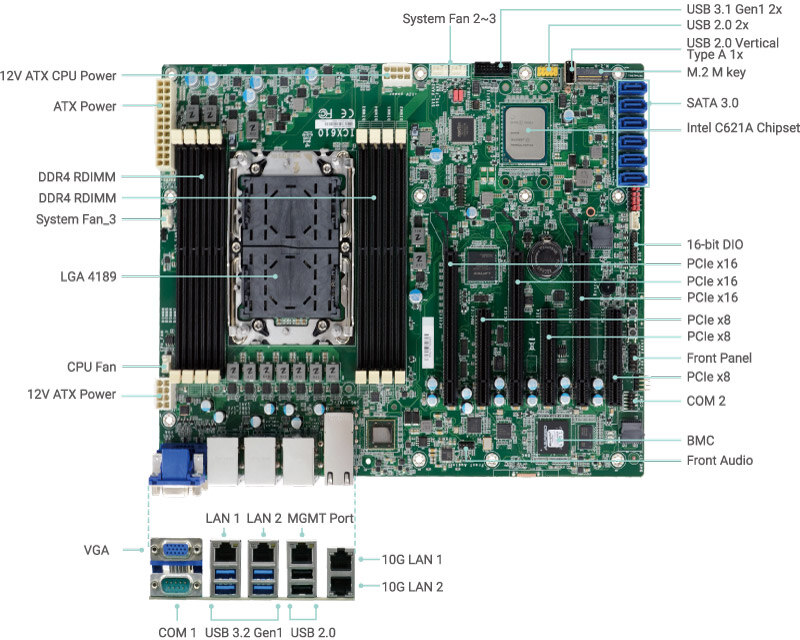
DFI server product line simplifies AIoT architecture in edge computing node deployment
With the increasing complexity of Internet of Things deployments, the amount of data received is becoming more diverse and the amount of information that needs to be processed is unparalleled, the DFI server product line simplifies the deployment of the Internet of Things with performance, high reliability and abundant bandwidth, reducing the difficulty of node deployment and increasing productivity while saving costs.
Download success story